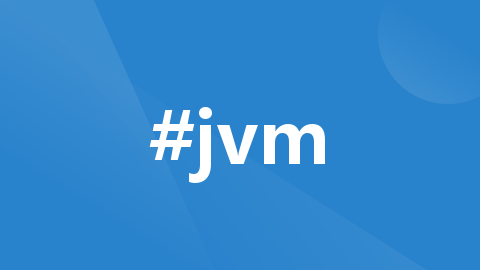
使用LlamaIndex实现SQL与向量数据库的路由查询引擎
"用于将自然语言查询转换为SQL查询,适用于city_stats表,包含每个城市的人口和国家信息"),description=f"用于回答关于。
·
在本篇文章中,我们将介绍如何使用LlamaIndex构建一个可以路由到SQL数据库或向量数据库的自定义查询引擎。我们将通过一个具体的示例展示如何进行设置、数据加载、构建索引以及定义查询引擎。为了适应国内用户访问,我们将使用中专API地址进行调用。
环境设置
首先,确保安装了所需的Python包:
%pip install llama-index
%pip install wikipedia
在Jupyter Notebook中,需要添加以下代码来解决事件循环问题:
import nest_asyncio
nest_asyncio.apply()
接下来,导入所需的库:
import logging
import sys
from sqlalchemy import create_engine, MetaData, Table, Column, String, Integer, insert
from llama_index.core import VectorStoreIndex, SQLDatabase
from llama_index.readers.wikipedia import WikipediaReader
from llama_index.core.query_engine import NLSQLTableQueryEngine, RouterQueryEngine
from llama_index.core.selectors import LLMSingleSelector
from llama_index.core.tools import QueryEngineTool
logging.basicConfig(stream=sys.stdout, level=logging.INFO)
logging.getLogger().addHandler(logging.StreamHandler(stream=sys.stdout))
创建数据库模式和测试数据
首先,我们创建一个SQLite数据库并添加一个示例表和数据:
# 创建SQLite数据库
engine = create_engine("sqlite:///:memory:", future=True)
metadata_obj = MetaData()
# 创建city_stats表
city_stats_table = Table(
"city_stats",
metadata_obj,
Column("city_name", String(16), primary_key=True),
Column("population", Integer),
Column("country", String(16), nullable=False),
)
metadata_obj.create_all(engine)
# 插入测试数据
rows = [
{"city_name": "Toronto", "population": 2930000, "country": "Canada"},
{"city_name": "Tokyo", "population": 13960000, "country": "Japan"},
{"city_name": "Berlin", "population": 3645000, "country": "Germany"},
]
for row in rows:
stmt = insert(city_stats_table).values(**row)
with engine.begin() as connection:
connection.execute(stmt)
加载数据
接下来,我们将从维基百科加载一些城市的数据,并创建向量索引:
# 安装维基百科包
!pip install wikipedia
# 加载维基百科数据
cities = ["Toronto", "Berlin", "Tokyo"]
wiki_docs = WikipediaReader().load_data(pages=cities)
# 为每个城市创建向量索引
vector_indices = []
for wiki_doc in wiki_docs:
vector_index = VectorStoreIndex.from_documents([wiki_doc])
vector_indices.append(vector_index)
构建SQL索引
我们将创建一个SQL索引以支持自然语言查询:
sql_database = SQLDatabase(engine, include_tables=["city_stats"])
sql_query_engine = NLSQLTableQueryEngine(
sql_database=sql_database,
tables=["city_stats"],
)
定义查询引擎并设置工具
我们将定义查询引擎并将其设置为工具,以便路由查询:
vector_query_engines = [index.as_query_engine() for index in vector_indices]
sql_tool = QueryEngineTool.from_defaults(
query_engine=sql_query_engine,
description=(
"用于将自然语言查询转换为SQL查询,适用于city_stats表,包含每个城市的人口和国家信息"
),
)
vector_tools = []
for city, query_engine in zip(cities, vector_query_engines):
vector_tool = QueryEngineTool.from_defaults(
query_engine=query_engine,
description=f"用于回答关于{city}的语义问题",
)
vector_tools.append(vector_tool)
定义路由查询引擎
最后,我们定义一个路由查询引擎来选择合适的工具处理查询:
query_engine = RouterQueryEngine(
selector=LLMSingleSelector.from_defaults(),
query_engine_tools=([sql_tool] + vector_tools),
)
response = query_engine.query("Which city has the highest population?")
print(str(response))
示例查询
我们可以进行一些示例查询:
response = query_engine.query("Tell me about the historical museums in Berlin")
print(str(response))
response = query_engine.query("Which countries are each city from?")
print(str(response))
可能遇到的错误
- 数据库连接失败: 确保数据库连接字符串正确且数据库服务器运行正常。
- 模块未安装: 确保所有必要的Python包已经安装,可以使用
pip install
命令进行安装。 - 查询语法错误: 自然语言查询可能会转换成错误的SQL语句,需检查查询引擎的配置和实现。
如果你觉得这篇文章对你有帮助,请点赞,关注我的博客,谢谢!
参考资料:
更多推荐
所有评论(0)