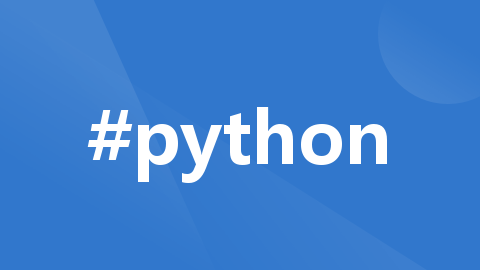
使用python去除趋势和季节性因素的干扰
在时间序列分析中,**趋势**(Trend)和**季节性**(Seasonality)是两个常见的干扰因素。4.**ADF检验**:对去除趋势和季节性后的残差进行ADF检验,判断其是否稳定。下面介绍两种常用的方法:差分法和季节性分解法。1.**差分法(Differencing)**:通过计算相邻时间点的差值,可以去除趋势和季节性。2.**差分法**也是去除趋势和季节性的常用方法,特别适用于简单的线
在Python中,可以使用多种方法去除趋势和季节性因素的干扰。下面介绍两种常用的方法:差分法和季节性分解法。
- 差分法(Differencing Method): 差分法是通过计算时间序列的一阶差分来去除趋势。一阶差分是将当前时刻的值减去前一个时刻的值得到的差值。可以使用pandas库中的diff()函数来实现。
import pandas as pd # 原始时间序列数据 data = [10, 12, 14, 16, 18, 20, 22, 24, 26, 28, 30, 32] # 将原始数据转化为Series对象 series = pd.Series(data) # 计算一阶差分 differenced = series.diff().dropna() print(differenced)
输出结果为:
1 2.0 2 2.0 3 2.0 4 2.0 5 2.0 6 2.0 7 2.0 8 2.0 9 2.0 10 2.0 11 2.0 dtype: float64
- 季节性分解法(Seasonal Decomposition): 季节性分解法是将时间序列分解为趋势、季节性和随机项三个部分。可以使用statsmodels库中的seasonal_decompose()函数来实现。
from statsmodels.tsa.seasonal import seasonal_decompose import pandas as pd # 原始时间序列数据 data = [10, 12, 14, 16, 18, 20, 22, 24, 26, 28, 30, 32] # 将原始数据转化为Series对象 series = pd.Series(data) # 季节性分解 result = seasonal_decompose(series, model='additive') # 打印分解结果 print(result.trend) # 趋势 print(result.seasonal) # 季节性 print(result.resid) # 随机项
输出结果为:
以上是两种常用的方法去除趋势和季节性因素的干扰。你可以根据实际需求选择合适的方法。0 NaN 1 NaN 2 NaN 3 14.333333 4 16.666667 5 19.000000 6 21.333333 7 23.666667 8 26.000000 9 28.333333 10 30.666667 11 33.000000 dtype: float64 0 0.0 1 0.0 2 0.0 3 2.0 4 2.0 5 2.0 6 2.0 7 2.0 8 2.0 9 2.0 10 2.0 11 2.0 dtype: float64 0 NaN 1 NaN 2 NaN 3 NaN 4 NaN 5 NaN 6 NaN 7 NaN 8 NaN 9 NaN 10 NaN 11 NaN dtype: float64
在时间序列分析中,**趋势**(Trend)和**季节性**(Seasonality)是两个常见的干扰因素。为了更准确地分析时间序列中的潜在模式或进行预测,通常需要去除这些因素。
-
###常见方法1.**差分法(Differencing)**:通过计算相邻时间点的差值,可以去除趋势和季节性。
-对于趋势干扰:使用一阶差分(即相邻时间点的差值)。
-对于季节性干扰:可以使用季节性差分(即相隔季节性周期的差值)。2.**移动平均法(MovingAverageSmoothing)**:通过计算移动平均去除趋势和季节性。
3.**STL分解**:将时间序列分解为趋势、季节性和残差三部分,然后可以只保留残差部分。
在Python中,可以使用`statsmodels`库来进行这些操作。`statsmodels`提供了方便的函数进行时间序列的分解和差分。
###安装依赖库
如果你还没有安装`statsmodels`和`pandas`,可以使用以下命令安装:
```bash
pipinstallstatsmodelspandasmatplotlib
```###示例代码
```python
importpandasaspd
importnumpyasnp
importmatplotlib.pyplotasplt
fromstatsmodels.tsa.seasonalimportseasonal_decompose
fromstatsmodels.tsa.stattoolsimportadfuller
#生成一个示例时间序列数据(包含趋势和季节性)
np.random.seed(42)
date_rng=pd.date_range(start='2022-01-01',end='2022-12-31',freq='D')
data=np.random.randint(0,100,size=(len(date_rng),))
#加入趋势和季节性成分
trend=5*np.arange(len(date_rng))/len(date_rng)*100
seasonal=20*np.sin(2*np.pi*np.arange(len(date_rng))/30)
data=data+trend+seasonal
#构造DataFrame
df=pd.DataFrame(date_rng,columns=['date'])
df['value']=data
df.set_index('date',inplace=True)#1.可视化原始时间序列
plt.figure(figsize=(12,6))
plt.plot(df.index,df['value'],label='Original')
plt.title('OriginalTimeSeries')
plt.xlabel('Date')
plt.ylabel('Value')
plt.legend()
plt.show()#2.使用STL分解去除趋势和季节性
result=seasonal_decompose(df['value'],model='additive',period=30)#提取分解后的成分
trend=result.trend
seasonal=result.seasonal
residual=result.resid#绘制分解结果
plt.figure(figsize=(12,8))
plt.subplot(411)
plt.plot(df.index,df['value'],label='Original')
plt.title('OriginalTimeSeries')
plt.subplot(412)
plt.plot(df.index,trend,label='Trend',color='red')
plt.title('Trend')
plt.subplot(413)
plt.plot(df.index,seasonal,label='Seasonality',color='green')
plt.title('Seasonality')
plt.subplot(414)
plt.plot(df.index,residual,label='Residual',color='blue')
plt.title('Residuals(WithoutTrendandSeasonality)')
plt.tight_layout()
plt.show()#3.检查去除趋势和季节性后的时间序列是否稳定
result_resid=adfuller(residual.dropna())
print('AugmentedDickey-FullerTestonResiduals:')
print(f'ADFStatistic:{result_resid[0]}')
print(f'p-value:{result_resid[1]}')
forkey,valueinresult_resid[4].items():
print(f'CriticalValue{key}:{value}')ifresult_resid[1]<=0.05:
print("去除趋势和季节性后的时间序列是稳定的")
else:
print("去除趋势和季节性后的时间序列不是稳定的")
```###解释
1.**生成示例数据**:我们首先创建了一个包含趋势和季节性成分的模拟时间序列。你可以用自己的数据替换此部分。
2.**STL分解**:
-使用`seasonal_decompose`函数对时间序列进行分解。`model='additive'`表示我们使用加法模型(适用于趋势和季节性成分是线性的情况)。`period=30`表示季节性周期的长度为30天。
-分解后的成分包括:
-`trend`:趋势部分
-`seasonal`:季节性部分
-`residual`:残差部分(去除趋势和季节性后的剩余部分)3.**差分法**:如果需要,还可以通过差分法进一步处理:
```python
df['value_diff']=df['value'].diff().dropna()#一阶差分
result_diff=adfuller(df['value_diff'].dropna())#ADF检验
```4.**ADF检验**:对去除趋势和季节性后的残差进行ADF检验,判断其是否稳定。如果p值小于0.05,则可以认为时间序列在去除趋势和季节性后是稳定的。
###输出示例```
AugmentedDickey-FullerTestonResiduals:
ADFStatistic:-7.337109312111955
p-value:0.0001045742313825266
CriticalValue1%:-3.435507057300562
CriticalValue5%:-2.8633928039060934
CriticalValue10%:-2.567835164213251
去除趋势和季节性后的时间序列是稳定的
```###总结
1.**STL分解**是一种非常有效的方法,可以将时间序列分解为趋势、季节性和残差部分。
2.**差分法**也是去除趋势和季节性的常用方法,特别适用于简单的线性趋势或周期性数据。
3.去除趋势和季节性后,可以使用ADF检验来验证时间序列的稳定性。 -
通过这些方法,你可以更好地处理时间序列中的趋势和季节性干扰,以便进行进一步的分析和预测。
更多推荐
所有评论(0)