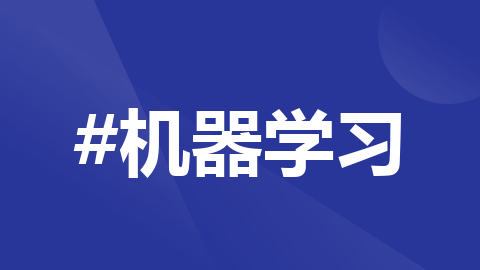
十大集成学习模型(简单版)
Bagging 通过在原始数据集的随机子集上训练多个基本模型,并对它们的预测结果进行平均或投票来减少方差。
前面介绍了:Ensemble Learning—集成学习-CSDN博客
10种最常见的集成算法模型,以及一些简单总结。
Bagging(自举聚合)
Boosting(提升)
Stacking(堆叠)
Blending(混合)
Voting(投票)
Bayesian Model Averaging(贝叶斯模型平均)
Gradient Tree Boosting with Randomness(GTBR)
Bayesian Additive Regression Trees(BART)
Rotation Forest
Negative Correlation Learning
在实际应用中,选择合适的集成学习算法需要考虑数据的特点、问题的复杂度以及计算资源等因素。
一、Bagging(自举聚合)
介绍:Bagging 通过在原始数据集的随机子集上训练多个基本模型,并对它们的预测结果进行平均或投票来减少方差。
核心点:每个基本模型是在独立的子集上训练的,这些子集通过自助采样(Bootstrap Sampling)得到。最终的预测结果是基于所有模型的平均或投票。
代码案例:
from sklearn.ensemble import BaggingClassifier
from sklearn.tree import DecisionTreeClassifier
from sklearn.datasets import make_classification
from sklearn.model_selection import train_test_split
from sklearn.metrics import accuracy_score
# 创建数据集
X, y = make_classification(n_samples=1000, n_features=20, random_state=42)
X_train, X_test, y_train, y_test = train_test_split(X, y, test_size=0.2, random_state=42)
# 创建基本分类器
base_classifier = DecisionTreeClassifier()
# 创建Bagging分类器
bagging_classifier = BaggingClassifier(base_estimator=base_classifier, n_estimators=10, random_state=42)
# 拟合模型
bagging_classifier.fit(X_train, y_train)
# 预测
y_pred = bagging_classifier.predict(X_test)
# 计算准确率
accuracy = accuracy_score(y_test, y_pred)
print("Bagging分类器的准确率:", accuracy)
二、Boosting(提升)
介绍:Boosting 通过训练一系列基本模型,每个模型都试图纠正前一个模型的错误,以提高预测性能。
核心点:每个基本模型的训练都依赖于前一个模型的性能,通常使用加权或梯度下降的方式来调整样本的权重,以便更关注错误分类的样本。
代码案例:
from sklearn.ensemble import AdaBoostClassifier
from sklearn.datasets import make_classification
from sklearn.model_selection import train_test_split
from sklearn.metrics import accuracy_score
# 创建数据集
X, y = make_classification(n_samples=1000, n_features=20, random_state=42)
X_train, X_test, y_train, y_test = train_test_split(X, y, test_size=0.2, random_state=42)
# 创建AdaBoost分类器
ada_classifier = AdaBoostClassifier(n_estimators=50, random_state=42)
# 拟合模型
ada_classifier.fit(X_train, y_train)
# 预测
y_pred = ada_classifier.predict(X_test)
# 计算准确率
accuracy = accuracy_score(y_test, y_pred)
print("AdaBoost分类器的准确率:", accuracy)
三、Stacking(堆叠)
介绍:Stacking 通过训练多个基本模型,并使用一个元模型来组合这些基本模型的预测结果,以提高整体性能。
核心点:首先,将训练数据集分成多个子集,用于训练不同的基本模型。然后,使用这些基本模型对测试集进行预测,并将这些预测结果作为新的特征。最后,使用元模型(通常是一个简单的线性模型)来学习这些新特征与真实标签之间的关系。
代码案例:
from sklearn.ensemble import RandomForestClassifier
from sklearn.ensemble import GradientBoostingClassifier
from sklearn.linear_model import LogisticRegression
from sklearn.model_selection import train_test_split
from sklearn.metrics import accuracy_score
# 创建数据集
X, y = make_classification(n_samples=1000, n_features=20, random_state=42)
X_train, X_test, y_train, y_test = train_test_split(X, y, test_size=0.2, random_state=42)
# 创建基本模型
base_model1 = RandomForestClassifier(n_estimators=50, random_state=42)
base_model2 = GradientBoostingClassifier(n_estimators=50, random_state=42)
# 拟合基本模型
base_model1.fit(X_train, y_train)
base_model2.fit(X_train, y_train)
# 使用基本模型进行预测
pred_model1 = base_model1.predict(X_test)
pred_model2 = base_model2.predict(X_test)
# 构建元特征
stacked_features = np.column_stack((pred_model1, pred_model2))
# 使用元模型
meta_model = LogisticRegression()
meta_model.fit(stacked_features, y_test)
# 结合预测结果
stacked_predictions = meta_model.predict(stacked_features)
# 计算准确率
accuracy = accuracy_score(y_test, stacked_predictions)
print("Stacking模型的准确率:", accuracy)
四、Blending(混合)
介绍:Blending是一种与Stacking类似的集成学习方法,区别在于Blending在训练阶段使用不同的数据集来训练基本模型,而不是直接使用全部训练数据。
核心点:Blending通常将训练数据集划分为两个部分,一个用于训练基本模型,另一个用于训练元模型。这种方法可以降低基本模型和元模型之间的过拟合风险。
代码案例:
# 分割训练数据集为两个子集
X_train_base, X_train_blend, y_train_base, y_train_blend = train_test_split(X_train, y_train, test_size=0.5, random_state=42)
# 训练基本模型
base_model1.fit(X_train_base, y_train_base)
base_model2.fit(X_train_base, y_train_base)
# 使用基本模型预测
pred_model1 = base_model1.predict(X_train_blend)
pred_model2 = base_model2.predict(X_train_blend)
# 构建元特征
blend_features = np.column_stack((pred_model1, pred_model2))
# 使用元模型
meta_model.fit(blend_features, y_train_blend)
# 在测试集上进行Blending预测
pred_model1_test = base_model1.predict(X_test)
pred_model2_test = base_model2.predict(X_test)
blend_features_test = np.column_stack((pred_model1_test, pred_model2_test))
blended_predictions = meta_model.predict(blend_features_test)
# 计算准确率
accuracy = accuracy_score(y_test, blended_predictions)
print("Blending模型的准确率:", accuracy)
五、Voting(投票)
介绍:Voting 通过组合多个基本模型的预测结果来做出最终的预测决策。可以分为硬投票和软投票两种方式。硬投票是基于多数投票原则,选择获得最多票数的类别作为最终预测结果;软投票则是基于预测概率的加权平均来进行投票。
核心点:Voting可以利用多个不同模型的优势,对不同模型的预测结果进行综合考量,从而得到更稳健和准确的预测结果。
代码案例:
from sklearn.ensemble import VotingClassifier
from sklearn.linear_model import LogisticRegression
from sklearn.svm import SVC
from sklearn.tree import DecisionTreeClassifier
from sklearn.datasets import make_classification
from sklearn.model_selection import train_test_split
from sklearn.metrics import accuracy_score
# 创建数据集
X, y = make_classification(n_samples=1000, n_features=20, random_state=42)
X_train, X_test, y_train, y_test = train_test_split(X, y, test_size=0.2, random_state=42)
# 创建基本分类器
logistic_clf = LogisticRegression(random_state=42)
svm_clf = SVC(probability=True, random_state=42)
tree_clf = DecisionTreeClassifier(random_state=42)
# 创建Voting分类器(硬投票)
voting_clf_hard = VotingClassifier(estimators=[('lr', logistic_clf), ('svm', svm_clf), ('tree', tree_clf)], voting='hard')
# 创建Voting分类器(软投票)
voting_clf_soft = VotingClassifier(estimators=[('lr', logistic_clf), ('svm', svm_clf), ('tree', tree_clf)], voting='soft')
# 拟合模型
voting_clf_hard.fit(X_train, y_train)
voting_clf_soft.fit(X_train, y_train)
# 预测
y_pred_hard = voting_clf_hard.predict(X_test)
y_pred_soft = voting_clf_soft.predict(X_test)
# 计算准确率
accuracy_hard = accuracy_score(y_test, y_pred_hard)
accuracy_soft = accuracy_score(y_test, y_pred_soft)
print("硬投票准确率:", accuracy_hard)
print("软投票准确率:", accuracy_soft)
六、Bayesian Model Averaging(贝叶斯模型平均)
介绍: 一种用来计算出多个模型权重的方法,贝叶斯模型平均利用贝叶斯方法将多个模型的预测结果进行加权平均,从而获得更加准确和稳健的预测结果。BMA 不仅考虑了各个模型的预测性能,还考虑了模型之间的不确定性,这使得 BMA 在处理多个模型的集成时更加灵活和健壮。
原理: BMA 的基本原理是基于贝叶斯定理和模型选择理论。在 BMA 中,首先假设有一个候选模型集合,每个模型都对数据生成过程做了不同的假设。然后,通过贝叶斯方法对每个模型的后验概率进行估计,即给定观测数据的情况下,对每个模型的预测能力进行评估。最后,利用模型的后验概率作为权重对模型的预测结果进行加权平均,得到最终的预测结果。
给定一个数据集 D和多个候选模型 ,目标是预测一个新数据点x 的结果 y。
核心公式:
通过上述公式,贝叶斯模型平均方法将多个模型的预测结果进行加权平均,以综合考虑每个模型的后验概率,从而得到更为稳健的预测结果。
代码案例:
import numpy as np
from sklearn.base import BaseEstimator, ClassifierMixin
from sklearn.metrics import log_loss
class BayesianModelAverageClassifier(BaseEstimator, ClassifierMixin):
def __init__(self, models):
self.models = models
def fit(self, X, y):
self.classes_ = np.unique(y)
self.probs_ = np.zeros((len(X), len(self.classes_)))
for model in self.models:
model.fit(X, y)
probs = model.predict_proba(X)
self.probs_ += probs
self.probs_ /= len(self.models)
def predict_proba(self, X):
return self.probs_
def predict(self, X):
return self.classes_[np.argmax(self.predict_proba(X), axis=1)]
# 使用示例
from sklearn.ensemble import RandomForestClassifier
from sklearn.naive_bayes import GaussianNB
from sklearn.datasets import make_classification
from sklearn.model_selection import train_test_split
from sklearn.metrics import accuracy_score
# 创建数据集
X, y = make_classification(n_samples=1000, n_features=20, random_state=42)
X_train, X_test, y_train, y_test = train_test_split(X, y, test_size=0.2, random_state=42)
# 创建模型集合
models = [
RandomForestClassifier(n_estimators=50, random_state=42),
GaussianNB()
]
# 创建 BMA 模型
bma_model = BayesianModelAverageClassifier(models)
# 拟合模型
bma_model.fit(X_train, y_train)
# 预测
y_pred = bma_model.predict(X_test)
# 计算准确率
accuracy = accuracy_score(y_test, y_pred)
print("BMA 模型的准确率:", accuracy)
七、Gradient Tree Boosting with Randomness (GTBR)
介绍:GTBR 是一种结合了梯度提升和随机性的集成学习方法。它通过在每个梯度提升迭代中引入随机性来提高模型的泛化性能。
核心点:GTBR 在每次迭代时,引入了随机性来构建弱学习器,例如在构建决策树时随机选择特征进行分割,或者对样本进行加权采样。这种随机性有助于减少模型的过拟合风险,并提高模型的泛化能力。
代码案例:GTBR 的典型实现包括 XGBoost、LightGBM 和 CatBoost。
import xgboost as xgb
from sklearn.datasets import make_classification
from sklearn.model_selection import train_test_split
from sklearn.metrics import accuracy_score
# 创建数据集
X, y = make_classification(n_samples=1000, n_features=20, random_state=42)
X_train, X_test, y_train, y_test = train_test_split(X, y, test_size=0.2, random_state=42)
# 创建XGBoost分类器
xgb_classifier = xgb.XGBClassifier(n_estimators=100, random_state=42)
# 拟合模型
xgb_classifier.fit(X_train, y_train)
# 预测
y_pred = xgb_classifier.predict(X_test)
# 计算准确率
accuracy = accuracy_score(y_test, y_pred)
print("XGBoost分类器的准确率:", accuracy)
八、Bayesian Additive Regression Trees (BART)
介绍:BART 是一种基于贝叶斯框架的非参数模型,通过将多个回归树模型进行组合来获得更好的预测性能。
核心点:BART 将多个回归树模型进行组合,每个回归树模型都被视为一个“基础学习器”。BART 使用马尔科夫链蒙特卡罗(MCMC)方法对模型参数进行估计,并通过组合多个回归树模型来获得更加灵活和鲁棒的预测结果。
代码案例:我们使用基于 MCMC 方法的贝叶斯回归树来近似实现 BART 模型的基本思想。
import numpy as np
from sklearn.tree import DecisionTreeRegressor
from sklearn.model_selection import train_test_split
from sklearn.metrics import mean_squared_error
class BayesianAdditiveRegressionTrees:
def __init__(self, n_trees=100, max_depth=3, alpha=1.0, beta=1.0):
self.n_trees = n_trees
self.max_depth = max_depth
self.alpha = alpha
self.beta = beta
self.trees = []
def fit(self, X, y):
self.trees = []
n_samples = X.shape[0]
for _ in range(self.n_trees):
tree = DecisionTreeRegressor(max_depth=self.max_depth)
idx = np.random.choice(n_samples, size=n_samples, replace=True)
tree.fit(X[idx], y[idx])
self.trees.append(tree)
def predict(self, X):
predictions = np.zeros(len(X))
for tree in self.trees:
predictions += tree.predict(X)
return predictions / self.n_trees
# 使用示例
# 创建模拟数据集
X = np.random.rand(100, 1) * 10
y = 2 * np.sin(X) + np.random.randn(100, 1)
# 划分训练集和测试集
X_train, X_test, y_train, y_test = train_test_split(X, y, test_size=0.2, random_state=42)
# 创建并拟合 BART 模型
bart_model = BayesianAdditiveRegressionTrees(n_trees=100, max_depth=3)
bart_model.fit(X_train, y_train)
# 预测
y_pred = bart_model.predict(X_test)
# 计算均方误差
mse = mean_squared_error(y_test, y_pred)
print("BART 模型的均方误差:", mse)
在这个示例中,定义了一个 BayesianAdditiveRegressionTrees 类来近似实现 BART 模型的基本思想。该类使用决策树作为基本模型,并通过随机抽样和平均的方式来构建多个树模型。
九、Rotation Forest
介绍:Rotation Forest 使用主成分分析(PCA)对输入特征进行变换,并在转换后的特征空间中训练多个基本分类器。
核心点 :Rotation Forest 的核心思想是通过主成分分析将原始特征空间转换为一个新的特征空间,然后在新的特征空间中训练多个基本分类器。这种方法可以在保留数据结构的同时,减少特征空间的维度,并提高分类性能。
代码案例:
from sklearn.pipeline import Pipeline
from sklearn.decomposition import PCA
from sklearn.ensemble import RandomForestClassifier
from sklearn.datasets import make_classification
from sklearn.model_selection import train_test_split
from sklearn.metrics import accuracy_score
# 创建数据集
X, y = make_classification(n_samples=1000, n_features=20, random_state=42)
X_train, X_test, y_train, y_test = train_test_split(X, y, test_size=0.2, random_state=42)
# 创建Rotation Forest模型
rotation_forest_model = Pipeline([
('pca', PCA(n_components=10)), # 使用PCA进行特征变换
('clf', RandomForestClassifier(n_estimators=100, random_state=42)) # 使用随机森林作为基本分类器
])
# 拟合模型
rotation_forest_model.fit(X_train, y_train)
# 预测
y_pred = rotation_forest_model.predict(X_test)
# 计算准确率
accuracy = accuracy_score(y_test, y_pred)
print("Rotation Forest模型的准确率:", accuracy)
十、Negative Correlation Learning
介绍: 负相关学习旨在通过确保集成模型中的个体学习器的预测结果具有负相关性来提高整体性能。这种方法旨在增加集成模型的多样性,减少模型之间的冗余性,从而提高模型的泛化能力。
原理: 负相关学习的核心原理是通过设计一种机制来确保个体学习器的预测结果之间具有负相关性。通常情况下,负相关学习通过以下步骤实现:
首先,训练多个基本学习器(如决策树、神经网络等)。
在每次集成时,对每个基本学习器的预测结果进行调整,以确保它们的相关性是负的。
将调整后的预测结果进行组合,得到最终的集成预测结果。
代码案例: 代码展示如何利用随机森林中的投票机制来实现负相关学习的思想。
from sklearn.ensemble import RandomForestClassifier
from sklearn.datasets import make_classification
from sklearn.model_selection import train_test_split
from sklearn.metrics import accuracy_score
# 创建数据集
X, y = make_classification(n_samples=1000, n_features=20, random_state=42)
X_train, X_test, y_train, y_test = train_test_split(X, y, test_size=0.2, random_state=42)
# 训练多个随机森林模型
n_estimators = 5
models = []
for _ in range(n_estimators):
model = RandomForestClassifier(n_estimators=10, random_state=42)
model.fit(X_train, y_train)
models.append(model)
# 组合预测结果
ensemble_predictions = []
for model in models:
predictions = model.predict(X_test)
ensemble_predictions.append(predictions)
# 计算负相关集成预测结果
negative_correlation_predictions = []
for i in range(len(X_test)):
ensemble_prediction = [predictions[i] for predictions in ensemble_predictions]
negative_correlation_prediction = max(set(ensemble_prediction), key=ensemble_prediction.count)
negative_correlation_predictions.append(negative_correlation_prediction)
# 计算准确率
accuracy = accuracy_score(y_test, negative_correlation_predictions)
print("负相关学习集成模型的准确率:", accuracy)
代码中,训练了多个随机森林模型,并利用它们的预测结果来构建负相关集成模型。具体地,采用了投票的方式,对多个模型的预测结果进行组合,以达到它们之间的相关性为负。
接下来学习:集成学习(一)Bagging-CSDN博客
更多推荐
所有评论(0)