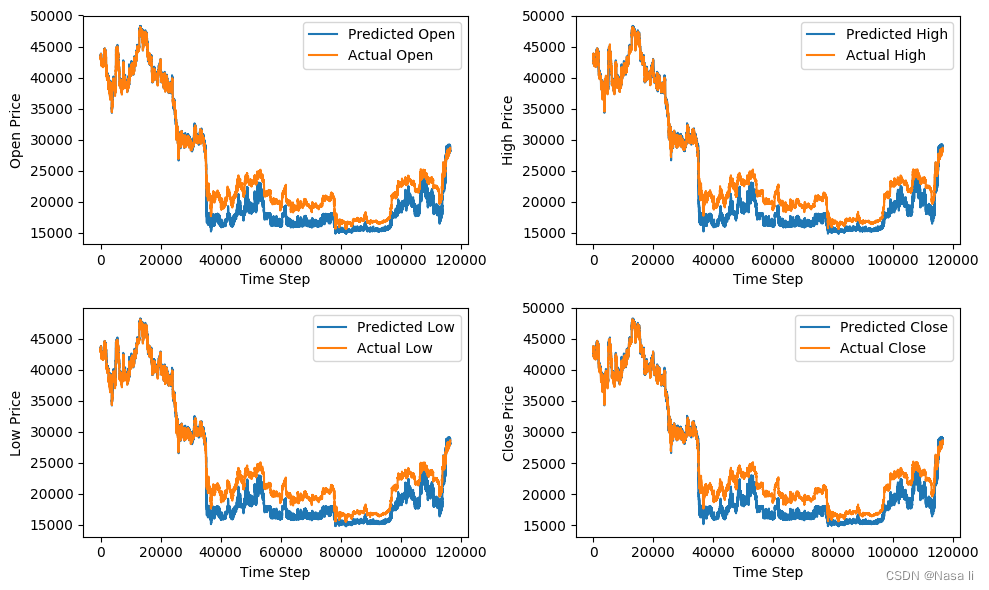
加密货币(btc)基于lstm的时间序列模型训练和预测
最近ai太火了,捡起以前比较喜欢的模型lstm对加密货币btc来了一波时间序列分析,期望是接入实时数据进行自动的量化交易,在这里分享我的经验欢迎更多的讨论和交流。
·
加密货币(btc)基于lstm的时间序列模型训练和预测
最近ai太火了,捡起以前比较喜欢的模型lstm对加密货币
btc
来了一波时间序列分析,期望是接入实时数据进行自动的量化交易,在这里分享我的经验欢迎更多的讨论和交流
数据获取
我选择了binance的交易数据(5分钟的k线),进行数据的获取数据已经开源在了百度paddlepaddle中,你可以点击paddle进行注册,数据地址位于公开的地址。
你也可以选择自己对接binance的api进行获取,项目已经开源在github欢迎start和issue,注意他们的api不支持中国大陆的服务器进行连接。
训练框架选择
选择了paddle作为模型代码的编写,他的接口目前用下来与pytoch
类似,有些细微的差别。之所以选择paddle是因为能够白嫖GPU进行模型训练。
项目大致思路
- 读取数据
- 数据新增特征(rsi、macd、ema、boll)这些特征是在交易中比较常使用到的,老韭菜应该知道
- 数据清洗
- 数据分割
- 模型定义
- 进行训练
- 模型预测
具体代码
import numpy as np
import pandas as pd
import paddle
from paddle import nn
from paddle.optimizer import Adam
from paddle.io import Dataset, DataLoader
from sklearn.preprocessing import MinMaxScaler
from sklearn.model_selection import train_test_split
import sqlite3
从数据库读取数据
# Connect to the SQLite database
connection = sqlite3.connect("./data/data203020/binance.db")
# Define the SQL query
sql_query = "SELECT * FROM BTCUSDT"
# Read data from the SQLite database into a pandas DataFrame
df = pd.read_sql_query(sql_query, connection)
# Close the database connection
connection.close()
修改数据结构
# 将字符串类型的数值列转换为浮点数
numeric_columns = ['open', 'high', 'low', 'close', 'volume', 'quote_asset_volume', 'taker_buy_base_asset_volume', 'taker_buy_quote_asset_volume']
df[numeric_columns] = df[numeric_columns].astype(float)
特征工程
# Calculate RSI
delta = df['close'].diff()
gain = delta.where(delta > 0, 0)
loss = -delta.where(delta < 0, 0)
avg_gain = gain.rolling(window=14).mean()
avg_loss = loss.rolling(window=14).mean()
rs = avg_gain / avg_loss
rsi = 100 - (100 / (1 + rs))
# Calculate EMA
ema_short = df['close'].ewm(span=12).mean()
ema_long = df['close'].ewm(span=26).mean()
# Calculate MACD
macd = ema_short - ema_long
signal_line = macd.ewm(span=9).mean()
histogram = macd - signal_line
# Add RSI, EMA, and MACD to the DataFrame
df['rsi'] = rsi
df['ema_short'] = ema_short
df['ema_long'] = ema_long
df['macd'] = macd
df['signal_line'] = signal_line
df['histogram'] = histogram
df['sma_3'] = df['close'].rolling(window=3).mean()
df['sma_6'] = df['close'].rolling(window=6).mean()
df['sma_12'] = df['close'].rolling(window=12).mean()
# 波动率
df['volatility_std'] = df['close'].rolling(window=5).std()
df['pct_change'] = df['close'].pct_change()
df['sma_20'] = df['close'].rolling(window=20).mean()
df['std_20'] = df['close'].rolling(window=20).std()
df['bollinger_upper'] = df['sma_20'] + 2 * df['std_20']
df['bollinger_middle'] = df['sma_20']
df['bollinger_lower'] = df['sma_20'] - 2 * df['std_20']
df['diff_bollinger_upper'] = df['close'] - df['bollinger_upper']
df['diff_bollinger_lower'] = df['close'] - df['bollinger_lower']
df['diff_sma_3'] = df['close'] - df['sma_3']
df['diff_sma_6'] = df['close'] - df['sma_6']
df['diff_sma_12'] = df['close'] - df['sma_12']
df.head()
open_time | open | high | low | close | volume | close_time | quote_asset_volume | number_of_trades | taker_buy_base_asset_volume | ... | sma_20 | std_20 | bollinger_upper | bollinger_middle | bollinger_lower | diff_bollinger_upper | diff_bollinger_lower | diff_sma_3 | diff_sma_6 | diff_sma_12 | |
---|---|---|---|---|---|---|---|---|---|---|---|---|---|---|---|---|---|---|---|---|---|
0 | 1504254000000 | 4716.47 | 4730.00 | 4716.47 | 4727.96 | 2.455540 | 1504254299999 | 11600.812852 | 32 | 1.659262 | ... | NaN | NaN | NaN | NaN | NaN | NaN | NaN | NaN | NaN | NaN |
1 | 1504254300000 | 4728.02 | 4762.99 | 4728.02 | 4762.99 | 1.969549 | 1504254599999 | 9375.300274 | 18 | 1.683266 | ... | NaN | NaN | NaN | NaN | NaN | NaN | NaN | NaN | NaN | NaN |
2 | 1504254600000 | 4731.12 | 4731.36 | 4731.12 | 4731.36 | 0.299831 | 1504254899999 | 1418.597288 | 3 | 0.000000 | ... | NaN | NaN | NaN | NaN | NaN | NaN | NaN | -9.410000 | NaN | NaN |
3 | 1504254900000 | 4750.00 | 4750.00 | 4746.63 | 4746.65 | 1.809314 | 1504255199999 | 8591.265774 | 13 | 0.983768 | ... | NaN | NaN | NaN | NaN | NaN | NaN | NaN | -0.350000 | NaN | NaN |
4 | 1504255200000 | 4746.65 | 4750.00 | 4746.65 | 4749.63 | 2.505225 | 1504255499999 | 11898.643549 | 22 | 1.041106 | ... | NaN | NaN | NaN | NaN | NaN | NaN | NaN | 7.083333 | NaN | NaN |
5 rows × 33 columns
df.columns
Index(['open_time', 'open', 'high', 'low', 'close', 'volume', 'close_time',
'quote_asset_volume', 'number_of_trades', 'taker_buy_base_asset_volume',
'taker_buy_quote_asset_volume', 'ignore', 'rsi', 'ema_short',
'ema_long', 'macd', 'signal_line', 'histogram', 'sma_3', 'sma_6',
'sma_12', 'volatility_std', 'pct_change', 'sma_20', 'std_20',
'bollinger_upper', 'bollinger_middle', 'bollinger_lower',
'diff_bollinger_upper', 'diff_bollinger_lower', 'diff_sma_3',
'diff_sma_6', 'diff_sma_12'],
dtype='object')
去除无用的字段ignore
data = df[['open_time', 'open', 'high', 'low', 'close', 'volume', 'close_time',
'quote_asset_volume', 'number_of_trades', 'taker_buy_base_asset_volume',
'taker_buy_quote_asset_volume', 'rsi', 'ema_short',
'ema_long', 'macd', 'signal_line', 'histogram', 'sma_3', 'sma_6',
'sma_12', 'volatility_std', 'pct_change', 'sma_20', 'std_20',
'bollinger_upper', 'bollinger_middle', 'bollinger_lower',
'diff_bollinger_upper', 'diff_bollinger_lower', 'diff_sma_3',
'diff_sma_6', 'diff_sma_12']]
数据清洗
去除nan
值
# 丢弃前20行含有nan的数据
data = data.iloc[20:]
data.reset_index(drop=True, inplace=True)
data.isna().sum()
data = data.fillna(0)
data.isna().sum()
数据分割,考虑时序数据的特征
数据分割需求
- 看了前n轮的数据预测n+1轮
scaler = MinMaxScaler()
data_scaled = scaler.fit_transform(data)
# 创建滑动窗口
def create_sliding_window(data, window_size):
x, y = [], []
for i in range(len(data) - window_size-1):
x.append(data[i:i+window_size, :])
y.append(data[i+window_size+1, 1:5]) # 第1行、第5列是因为需要的是只有5个属性
return np.array(x), np.array(y)
# 设定窗口大小(例如:30个时间步长)
window_size = 30
X, y = create_sliding_window(data_scaled, window_size)
# 数据划分
train_ratio = 0.8
train_size = int(len(X) * train_ratio)
X_train, X_test = X[:train_size], X[train_size:]
y_train, y_test = y[:train_size], y[train_size:]
# 将数据转换为PaddlePaddle所需的格式
X_train, y_train = paddle.to_tensor(X_train).astype('float32'), paddle.to_tensor(y_train).astype('float32')
X_test, y_test = paddle.to_tensor(X_test).astype('float32'), paddle.to_tensor(y_test).astype('float32')
W0419 10:19:31.641144 182 gpu_resources.cc:61] Please NOTE: device: 0, GPU Compute Capability: 8.0, Driver API Version: 11.2, Runtime API Version: 11.2
W0419 10:19:31.644184 182 gpu_resources.cc:91] device: 0, cuDNN Version: 8.2.
构建模型结构
# 构建自定义模型
class CustomModel(nn.Layer):
def __init__(self, input_size, hidden_size, output_size):
super(CustomModel, self).__init__()
self.bidirectional_lstm1 = nn.LSTM(input_size, hidden_size, direction="bidirectional")
self.bidirectional_lstm2 = nn.LSTM(hidden_size * 2, hidden_size, direction="bidirectional")
self.pooling = nn.AdaptiveAvgPool1D(1)
self.unidirectional_lstm = nn.LSTM(hidden_size * 2, hidden_size, dropout=0.041)
self.fc = nn.Linear(hidden_size, output_size)
def forward(self, x):
x, _ = self.bidirectional_lstm1(x)
x, _ = self.bidirectional_lstm2(x)
x = self.pooling(x.transpose([0, 2, 1])).transpose([0, 2, 1])
x, _ = self.unidirectional_lstm(x)
x = self.fc(x[:, -1, :])
return x
设置模型参数
# 模型参数设置
input_size = X_train.shape[2]
hidden_size = 166
output_size = 4
# 初始化模型、损失函数、优化器
model = CustomModel(input_size, hidden_size, output_size)
loss_fn = nn.MSELoss()
optimizer = paddle.optimizer.Adam(learning_rate=0.001, parameters=model.parameters())
开始训练,并且保存模型
经过多次训练,模型在200轮后代价函数不再降低,选择训练到300轮停止训练
# 训练参数设置
epochs = 2000
batch_size = 512
import os
# 定义模型保存路径
model_save_dir = 'saved_models'
if not os.path.exists(model_save_dir):
os.makedirs(model_save_dir)
# 训练模型
for epoch in range(epochs):
for i in range(0, len(X_train), batch_size):
X_batch = X_train[i:i+batch_size]
y_batch = y_train[i:i+batch_size]
y_pred = model(X_batch)
loss = loss_fn(y_pred, y_batch)
loss.backward()
optimizer.step()
optimizer.clear_grad()
print(f'Epoch {epoch + 1}, Loss: {loss.numpy()[0]}')
# 每100轮保存一次模型
if (epoch + 1) % 100 == 0:
model_path = os.path.join(model_save_dir, f'epoch_{epoch + 1}_model.pdparams')
paddle.save(model.state_dict(), model_path)
print(f'Model saved at epoch {epoch + 1}: {model_path}')
Epoch 1, Loss: 0.013917500153183937
Epoch 2, Loss: 0.007578677032142878
KeyboardInterrupt:
使用模型进行测试
# 加载模型
model = CustomModel(input_size, hidden_size, output_size)
model_state_dict = paddle.load('saved_models/epoch_1900_model.pdparams')
model.load_dict(model_state_dict)
model.eval()
# 使用测试数据进行预测
# y_pred = model(X_test) 太大了GPU不够
batch_size = 512
n_batches = int(np.ceil(X_test.shape[0] / batch_size))
y_pred_list = []
for i in range(n_batches):
start_idx = i * batch_size
end_idx = min((i + 1) * batch_size, X_test.shape[0])
X_test_batch = X_test[start_idx:end_idx]
with paddle.no_grad():
y_pred_batch = model(X_test_batch)
y_pred_list.append(y_pred_batch.numpy())
# y_pred_batch = model(X_test[0:30])
# y_pred_batch.numpy()
# 将预测结果转换为Numpy数组
y_pred_np = np.concatenate(y_pred_list, axis=0)
# 反归一化预测结果
y_test_np = y_test.numpy()
temp_test = np.zeros((y_test_np.shape[0], data_scaled.shape[1]))
temp_pred = np.zeros((y_pred_np.shape[0], data_scaled.shape[1]))
temp_test[:, 1:5] = y_test_np
temp_pred[:, 1:5] = y_pred_np
y_test_unscaled = scaler.inverse_transform(temp_test)[:, 1:5]
y_pred_unscaled = scaler.inverse_transform(temp_pred)[:, 1:5]
# 计算评估指标(如:RMSE)
from sklearn.metrics import mean_squared_error
rmse = np.sqrt(mean_squared_error(y_test_np, y_pred_np))
print(f'RMSE: {rmse}')
RMSE: 0.03624340891838074
# 可视化预测结果
import matplotlib.pyplot as plt
fig, axes = plt.subplots(2, 2, figsize=(10, 6))
axes[0, 0].plot(y_pred_unscaled[:, 0], label='Predicted Open')
axes[0, 0].plot(y_test_unscaled[:, 0], label='Actual Open')
axes[0, 0].set_xlabel('Time Step')
axes[0, 0].set_ylabel('Open Price')
axes[0, 0].legend()
axes[0, 1].plot(y_pred_unscaled[:, 1], label='Predicted High')
axes[0, 1].plot(y_test_unscaled[:, 1], label='Actual High')
axes[0, 1].set_xlabel('Time Step')
axes[0, 1].set_ylabel('High Price')
axes[0, 1].legend()
axes[1, 0].plot(y_pred_unscaled[:, 2], label='Predicted Low')
axes[1, 0].plot(y_test_unscaled[:, 2], label='Actual Low')
axes[1, 0].set_xlabel('Time Step')
axes[1, 0].set_ylabel('Low Price')
axes[1, 0].legend()
axes[1, 1].plot(y_pred_unscaled[:, 3], label='Predicted Close')
axes[1, 1].plot(y_test_unscaled[:, 3], label='Actual Close')
axes[1, 1].set_xlabel('Time Step')
axes[1, 1].set_ylabel('Close Price')
axes[1, 1].legend()
plt.tight_layout()
plt.show()
todo
- 模型转化为生产
- 接入实时数据
收盘价
# 可视化预测结果(仅收盘价)
plt.figure(figsize=(10, 6))
plt.plot(y_pred_unscaled[100000:100010, 3], label='Predicted Close')
plt.plot(y_test_unscaled[100000:100010, 3], label='Actual Close')
plt.xlabel('Time Step')
plt.ylabel('Close Price')
plt.legend()
plt.show()
声明
本文为nasa1024原创,如需转载请向lihangdemail1996@gmail.com 发送申请邮件,违规转载必究。
项目地址
https://github.com/nasa1024/Lying2EarnMoney欢迎讨论和issue
我的博客地址nasa’s space
更多推荐
所有评论(0)