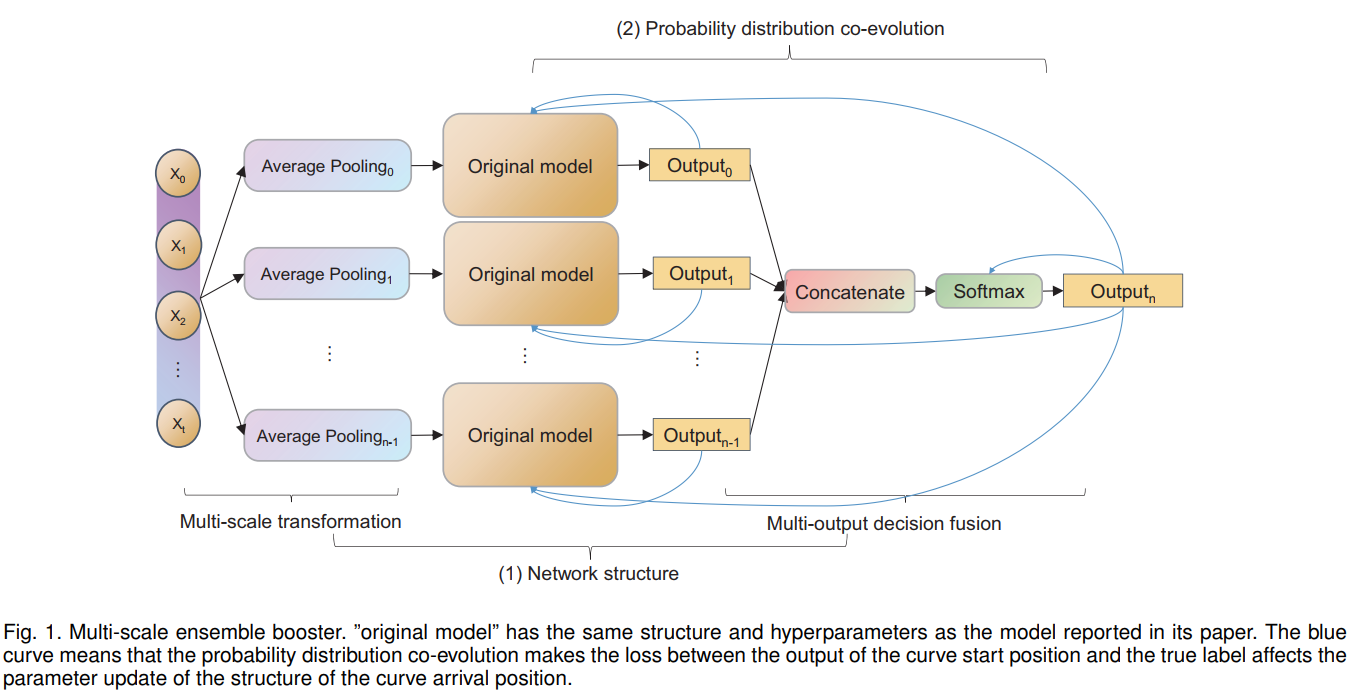
TKDE期刊2023.10 paper-提升现有时间序列数据分类器的多尺度集成增强器(实现最先进的时间序列数据分类性能)CCF A类 数据挖掘顶刊论文python代码收藏
我们提出了一种高度易于使用的性能增强框架,称为多尺度集成增强器(MEB),帮助现有的时间序列数据分类器实现性能飞跃。我们的实验证明了MEB的显著性能提升能力以及被MEB增强的模型可拥有最先进的时间序列数据分类性能。
以下代码链接是Multi-Scale Ensemble Booster for Improving Existing TSD Classifiers论文代码,包含了算法和表格等,比较完备。
论文:Multi-Scale Ensemble Booster for Improving Existing TSD Classifiers
论文代码github网址如下:点击绿色code,然后download zip。
#提升现有时间序列数据分类器的多尺度集成增强器
我们提出了一种高度易于使用的性能增强框架,称为多尺度集成增强器(MEB),帮助现有的时间序列数据分类器实现性能飞跃。此外,我们提出了一种新的度量方法,用于评估多数据集上方法的时间序列分类性能,称为MACE。
在MEB中,我们提出了一种易于组合的网络结构,无需改变其结构和超参数,只需设置一个超参数,由多尺度变换和多输出决策融合组成。然后,提出了一种概率分布协同进化策略,以获得最优的标签概率分布。我们在128个单变量数据集和29个多变量数据集上进行了多次MEB消融实验,并使用11种最先进的方法进行了对比实验,这分别证明了MEB的显著性能改善能力和MEB增强的模型的最先进性能。此外,为了弄清楚MEB为什么可以提高模型性能,我们提供了一系列可解释性分析。
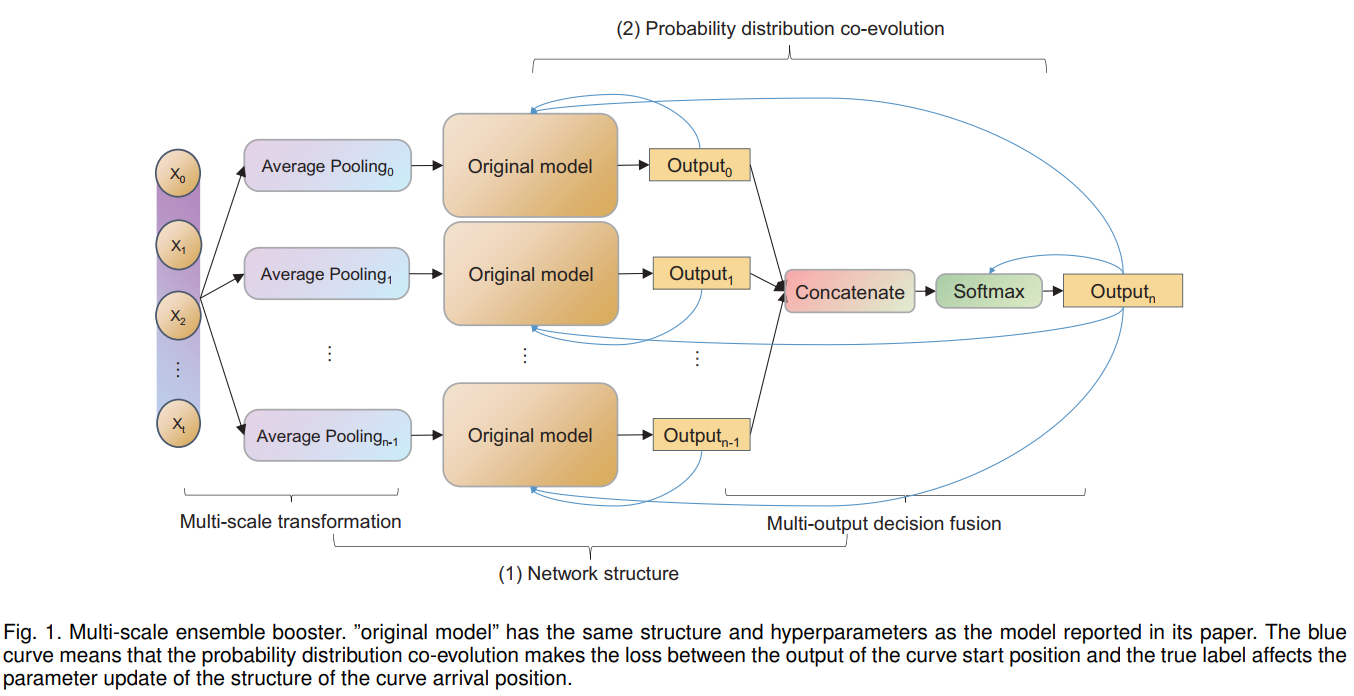
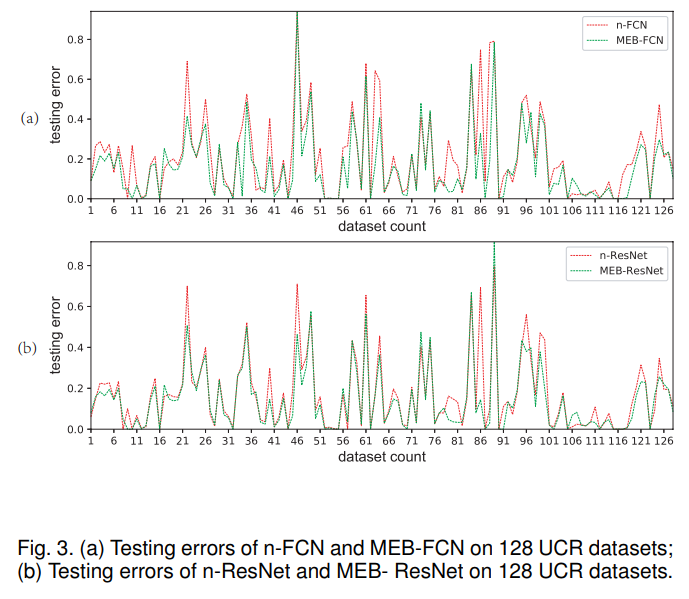
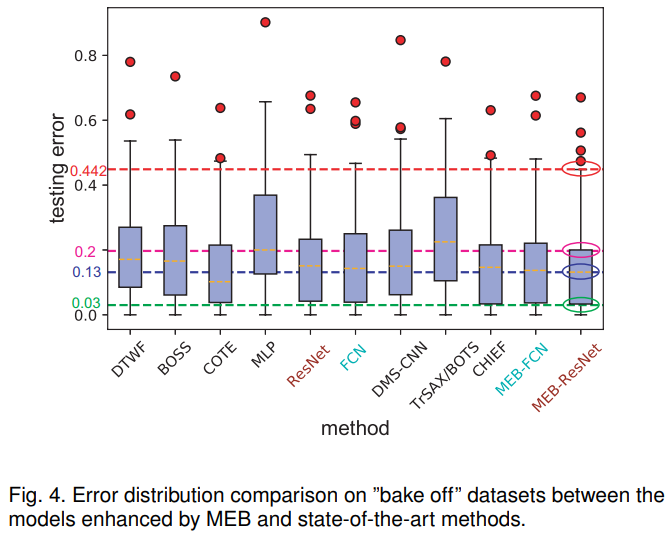
#易于成功运行
为了使代码易于成功运行,我们仔细调试文件。一般来说,如果环境得到满足,您可以在解压缩压缩包后直接运行其中的所有xxx.py文件,而无需更改任何代码。
(1) 解压缩Multi-Scale-Ensemble-Booster-for-Improveg-Existing-Time-Series-Data-Classifiers.zip。
(2) 直接运行任何xxx.py
数据集参考网站:Welcome to the UCR Time Series Classification/Clustering Page(UCR存储库:单变量时间序列数据集)和https://github.com/titu1994/MLSTM-FCN/releases(多变量时间序列数据集)
#文章与引用
(1) 为了更好地理解我们的代码,请阅读我们的论文。
论文:Multi-Scale Ensemble Booster for Improving Existing TSD Classifiers
(2) 出于学术目的使用代码时,请引用本文和数据集的原始来源。
BibTex:
@ARTICLE{multi2022fan,
author={Fan, Linchuan and Chai, Yi and Chen, Xiaolong},
journal={IEEE Transactions on Knowledge and Data Engineering},
title={Multi-Scale Ensemble Booster for Improving Existing TSD Classifiers},
year={2022},
volume={},
number={},
pages={1-17},
doi={10.1109/TKDE.2022.3230709}}
GB/T 7714:
Fan L, Chai Y, Chen X. Multi-Scale Ensemble Booster for Improving Existing TSD Classifiers[J]. IEEE Transactions on Knowledge and Data Engineering, 2022.
#代码与论文的关系
方法:
(1) MEB-ResNet: code\Ablation_experiment_on_128_UCR\MEB-ResNet_128_UCR.py
(2) MEB-FCN:code\Ablation_experiment_on_128_UCR\MEB-FCN_128_UCR.py
我们提出的指标,其用于评估一个方法在多个时序数据集上的性能:
(1) MACE: Section 4.1 : the def MACE(x1,num_classes) in code\table\Table_3_4_5_6.py
实验:
(1) Section 4.2.1. :code\Ablation_experiment_of_pooling_methods_on_MTSC_datasets
(2) Section 4.2.2 and 4.2.3. :code\Ablation_experiment_on_128_UCR and code\Ablation_and_comparision_experiment_on_MTSC_datasets
(3) Section 4.3. :code\Comparision_experiment_on_85_UCR and code\Ablation_and_comparision_experiment_on_MTSC_datasets
# 环境与致谢:
(1) 环境:
tensorflow-gpu 1.15.0
keras 2.2.4
scipy 1.5.2
pandas 1.0.5
numpy 1.19.1
(2) 致谢:
衷心感谢以下参考 .
github: https://github.com/cauchyturing/UCR_Time_Series_Classification_Deep_Learning_Baseline
github: https://github.com/titu1994/MLSTM-FCN/releases
UCR:Welcome to the UCR Time Series Classification/Clustering Page
更多推荐
所有评论(0)