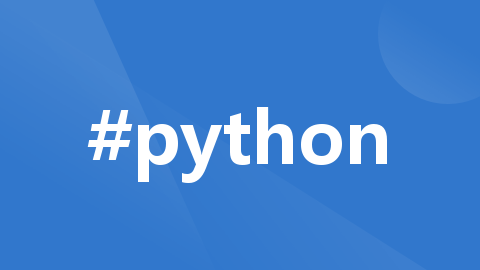
python:使用scipy及numpy对三维空间点进行插值并可视化
使用scipy及numpy对三维空间点进行插值并可视化
示例 1
要在Python中对三维坐标空间点进行插值,可以使用SciPy库中的插值函数。首先,需要安装SciPy库。可以使用以下命令来安装:
pip install scipy
接下来,可以使用以下代码对给定的三个点进行插值,并可视化插值前后的点:
import numpy as np
from scipy.interpolate import interp1d
import matplotlib.pyplot as plt
from mpl_toolkits.mplot3d import Axes3D
# 给定的三个点
points = np.array([[2.1, 4, 1], [3.2, 4.1, 1.5], [5.5, 3.8, 1.2]])
# 拆分三个轴的值
x = points[:, 0]
y = points[:, 1]
z = points[:, 2]
# 创建插值函数
f = interp1d(x, y)
# 生成插值后的新点
new_x = np.linspace(x.min(), x.max(), 100)
new_y = f(new_x)
new_z = np.interp(new_x, x, z)
interp_points = np.stack((new_x, new_y, new_z), 1)
# 绘制原始点
fig = plt.figure()
ax = fig.add_subplot(111, projection='3d')
ax.scatter(x, y, z, color='blue', label='Original Points')
# 绘制插值后的点
ax.scatter(new_x, new_y, new_z, color='red', label='Interpolated Points')
# 设置图形属性
ax.set_xlabel('X')
ax.set_ylabel('Y')
ax.set_zlabel('Z')
ax.legend()
plt.show()
以上代码首先导入了所需的库。接下来,定义了给定的三个点,并拆分成三个轴的值。然后,使用interp1d
函数创建了一个插值函数。最后,使用linspace
函数生成插值后的新点,并使用scatter
函数绘制了原始点和插值后的点。
运行以上代码,将显示一个包含原始点和插值后点的三维散点图。
示例 2
可以使用插值方法来生成更多的点。以下是一种方法:
-
计算已知点之间的距离。可以使用欧几里得距离公式,即:d = sqrt((x2-x1)^2 + (y2-y1)^2 + (z2-z1)^2)。
-
将已知点之间的距离平均分成若干段,确定插值点间的步长(step)。
-
以其中一个已知点为起始点,计算插值点的坐标。假设起始点坐标为(x1, y1, z1),步长为step,插值点的坐标可以通过以下公式计算:
- x = x1 + t * (x2 - x1)
- y = y1 + t * (y2 - y1)
- z = z1 + t * (z2 - z1)
其中,t为0到1之间的值,表示在已知点之间的位置。
-
重复步骤3,直到计算出所有插值点的坐标。
下面是一个示例代码,实现了上述方法:
import math
def interpolate_points(points, num_points):
interpolated_points = []
# 计算已知点之间的距离
distances = []
for i in range(len(points)-1):
x1, y1, z1 = points[i]
x2, y2, z2 = points[i+1]
distance = math.sqrt((x2-x1)**2 + (y2-y1)**2 + (z2-z1)**2)
distances.append(distance)
# 计算插值点的步长
total_distance = sum(distances)
step = total_distance / num_points
# 生成插值点
for i in range(len(points)-1):
x1, y1, z1 = points[i]
x2, y2, z2 = points[i+1]
distance = distances[i]
num_interpolated_points = int(distance / step)
for j in range(num_interpolated_points):
t = (j+1) / (num_interpolated_points+1)
x = x1 + t * (x2-x1)
y = y1 + t * (y2-y1)
z = z1 + t * (z2-z1)
interpolated_points.append((x, y, z))
return interpolated_points
# 示例用法
points = [(0, 0, 0), (1, 1, 1), (2, 2, 2), (3, 3, 3)]
num_points = 5
interpolated_points = interpolate_points(points, num_points)
for point in interpolated_points:
print(point)
在上面的示例中,已知三个点的坐标为(0, 0, 0),(1, 1, 1)和(2, 2, 2),要生成5个插值点。运行代码后,将会输出插值点的坐标。
示例 3
要在Python中根据已知的三个以上的三维空间点进行插值,您可以使用插值函数来生成更多的点。一种常用的方法是使用SciPy库中的scipy.interpolate模块。以下是一个示例代码,说明如何使用二次插值函数进行插值:
import numpy as np
from scipy.interpolate import griddata
# 已知的三维空间点
x = [1, 2, 3]
y = [4, 5, 6]
z = [7, 8, 9]
# 定义插值网格
xi = np.linspace(min(x), max(x), 10)
yi = np.linspace(min(y), max(y), 10)
# 生成网格坐标
xi, yi = np.meshgrid(xi, yi)
# 进行二次插值
zi = griddata((x, y), z, (xi, yi), method='cubic')
# 输出插值结果
print(zi)
在上述代码中,我们首先定义了三个已知的三维空间点的坐标分别为x、y和z。然后我们定义了插值的网格坐标xi和yi,可以通过调整这两个数组的大小来控制生成的插值点的数量。接下来,我们使用griddata
函数进行二次插值,指定了插值的数据点坐标(x, y)和对应的值z,以及插值网格的坐标(xi, yi)和插值方法’cubic’。最后,我们输出了插值结果zi。
请注意,插值方法有不同的选择,可以根据需要选择最合适的方法。此外,为了使用上述代码,您需要安装SciPy库。您可以使用命令pip install scipy
来安装SciPy库。
更多推荐
所有评论(0)