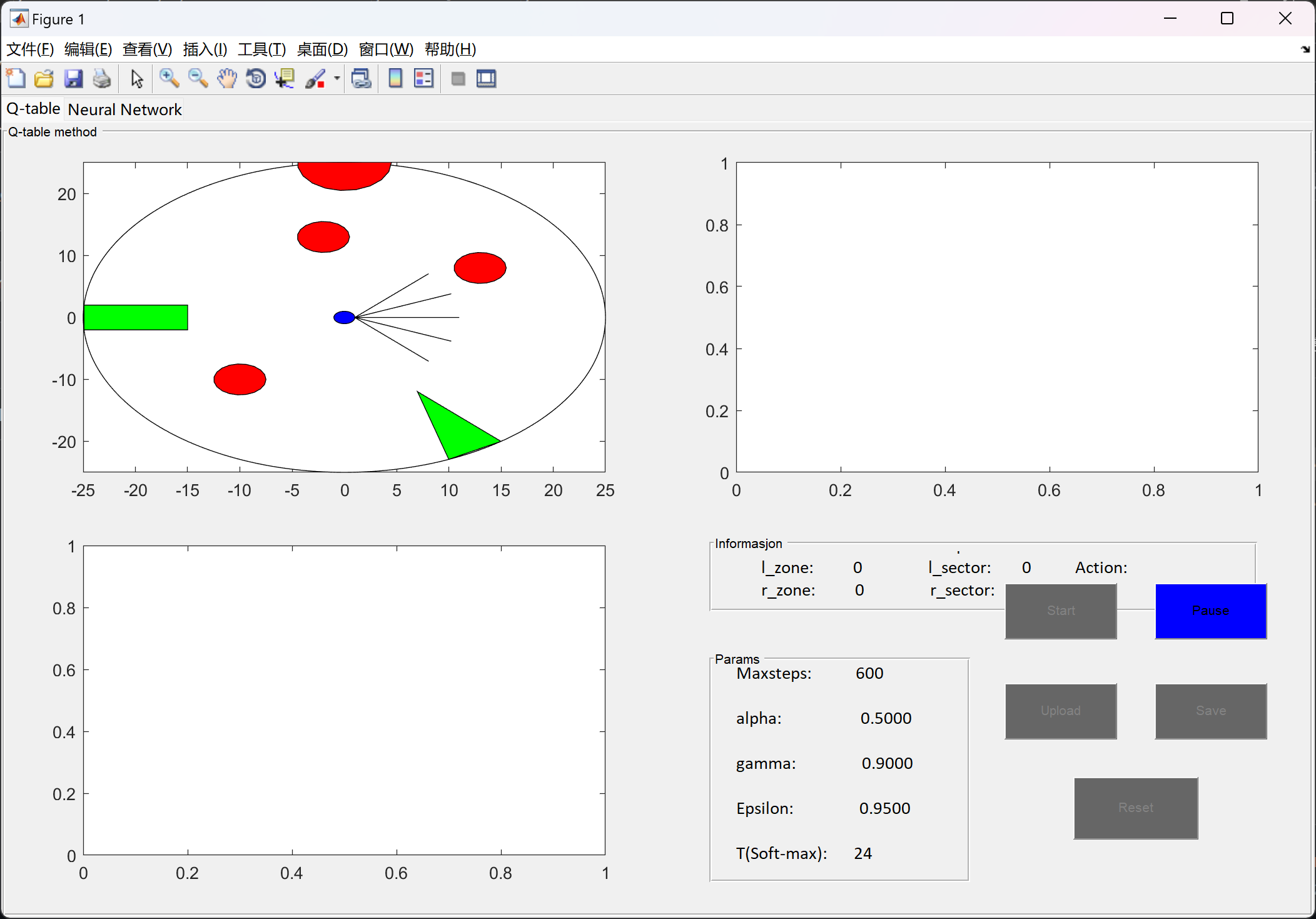
【GUI】强化学习与 Q-Learning(Q 学习)研究(Matlab代码实现)
尽管 Q-Learning 在简单的环境中表现良好,但在复杂的、大规模的环境中,它可能会受到状态空间和动作空间的爆炸性增长的限制。Q-Learning(Q 学习)是强化学习中的一种经典算法,它是基于值函数的一种迭代学习方法,用于学习在给定状态下采取特定动作的最优策略。总的来说,Q-Learning 是强化学习领域的一个重要里程碑,它为解决各种实际问题提供了一个简单而强大的框架,并且在理论研究和实际
💥💥💞💞欢迎来到本博客❤️❤️💥💥
🏆博主优势:🌞🌞🌞博客内容尽量做到思维缜密,逻辑清晰,为了方便读者。
⛳️座右铭:行百里者,半于九十。
📋📋📋本文目录如下:🎁🎁🎁
目录
💥1 概述
强化学习是一种机器学习范式,其主要目标是使智能体通过与环境的交互来学习如何在特定任务中获得最大的累积奖励。Q-Learning(Q 学习)是强化学习中的一种经典算法,它是基于值函数的一种迭代学习方法,用于学习在给定状态下采取特定动作的最优策略。
在 Q-Learning 中,智能体试图学习一个称为 Q 值函数的映射,它将状态-动作对映射到未来累积奖励的预期值。Q 值函数的更新过程基于贝尔曼方程,它表示当前状态-动作对的 Q 值应该等于即时奖励与下一状态的最大 Q 值的折扣之和。Q-Learning 通过在不断的探索和利用之间进行权衡,逐步更新 Q 值函数,以使其逼近最优策略。
Q-Learning 的核心思想是通过与环境的交互来积累经验,并根据这些经验不断更新 Q 值函数,最终使智能体能够在未知的环境中找到最优策略。尽管 Q-Learning 在简单的环境中表现良好,但在复杂的、大规模的环境中,它可能会受到状态空间和动作空间的爆炸性增长的限制。因此,对 Q-Learning 进行改进和扩展是当前研究的一个重要方向,例如使用深度学习方法结合强化学习(深度 Q 网络)来处理高维状态空间和动作空间的问题。
总的来说,Q-Learning 是强化学习领域的一个重要里程碑,它为解决各种实际问题提供了一个简单而强大的框架,并且在理论研究和实际应用中都有着广泛的影响和应用。
📚2 运行结果
部分代码:
n_crash1 = 0; % total number of crash with Q-table
n_crash2 = 0; % total number of crash with Nueral-Network
reward1 = 0; % reward of the Q-tabell
reward2 = 0;
total_reward1 = 0; % total rewad of the Q-table
total_reward2 = 0;
cost_fun = 0;
% Select between static and dynamic obstacles
% dynamic obstacles = 'Dynamic', static obstacles = 'Static'
stadium_option1 = 'Static';
stadium_option2 = 'Static';
% Properties of the vehicle. scale: 1:10 cm
radius = 1.0;
wheel_radius = 0.325;
sensor_lengde = 10;
tot_rot = 0;
%% ----- Initialize Q-learing with Q-table
state_space = createStateSpace(stadium_option1); % state space
actions = actionList(); % action list
%Find the size of the state space and the length of the action list
n_states = size(state_space,1);
n_actions = length(actions);
% Initialize Q-table and the model
Q_table = createQTable(n_states, n_actions); %Q-table
%% ----- Initialize Q-learning with Neural Network
%Neural Network
input_layer_size = 5;
hidden_layer_size = 15;
output_layer_size = 3;
initial_w1 = randInitializeWeights(input_layer_size, hidden_layer_size);
initial_w2 = randInitializeWeights(hidden_layer_size, output_layer_size);
nn_params = [initial_w1(:); initial_w2(:)];
%% ----- Initaliseringer av parametrer for Q-tabell og Neural Network metoden
%Initalisreringer av forskjellige parametrer
max_trials = 10000; % Max Trials
max_steps = 600; % Max steps
alpha1 = 0.5; % Learning rate
alpha2 = 0.03;
gamma = 0.9; % Discount factor
epsilon = 0.95; % Chance for random action
T1 = 24; % The Paramater of the explore function with blotzmanns distribution
T2 = 24;
%% ----- Initaliseringer for GUI
%Plottets st鴕relse
xlimit = [-25, 25];
ylimit = [-25, 25];
% GUI
[h_plot1, h_plot2, h_text1, h_text2, h_button1, h_button2] = createSimulation(xlimit, ylimit, max_trials, max_steps,...
alpha1, alpha2, gamma, epsilon, T1);
% Simulation Environments
🎉3 参考文献
文章中一些内容引自网络,会注明出处或引用为参考文献,难免有未尽之处,如有不妥,请随时联系删除。
[1]刘忠,李海红,刘全.强化学习算法研究[J].计算机工程与设计, 2008, 29(22):5.DOI:CNKI:SUN:SJSJ.0.2008-22-044.
[2]徐莉.Q-learning研究及其在AUV局部路径规划中的应用[D].哈尔滨工程大学,2004.DOI:10.7666/d.y670628.
[3]张汝波,杨广铭,顾国昌,等.Q-学习及其在智能机器人局部路径规划中的应用研究[J].计算机研究与发展, 1999, 36(12):7.DOI:10.1007/BF02946502.
🌈4 Matlab代码实现
更多推荐
所有评论(0)