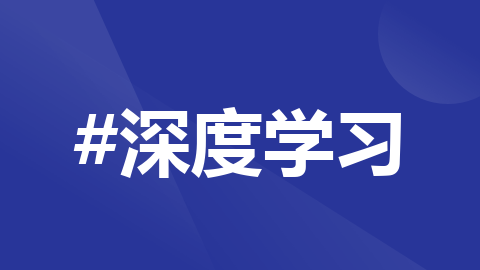
【深度学习】球衣号码识别 re-id追踪
此外,CLIP Vision Transformers 拥有强大的OCR能力,可以在对没有数据集进行任何微调的情况下,以zero-shot manner without any fine-tuning on the dataset。3.论文:CLIP-ReIdent: Contrastive Training for Player Re-Identificationhttps://arxiv.or
1. CLIP-ReIdent: Contrastive Training for Player Re-Identification 论文解析–2023的论文,貌似顶会
论文方法是类不可知的,微调CLIP vitl/14模型,在MMSports 2022球员重新识别挑战中实现98.44%的mAP。此外,CLIP Vision Transformers 拥有强大的OCR能力,可以在对没有数据集进行任何微调的情况下,以zero-shot manner without any fine-tuning on the dataset。通过应用score-scam算法,可以可视化最重要的图像区域。计算两张球员图片相似性得分时识别这些区域。
原来的RID存在的问题:侧重于多视图依赖特征,衣服变化和野外识别和球员的rid识别有很大不同。
基于player tracking 和 reidentification的运动分析来分项一个运动员的性能。
球员识别和行人识别的区别:1.同质化背景,篮球场、冰球场标准化场地。2.穿戴相同。
因此,号码、鞋、脸很重要。
困难点:图像低分辨率可能动态模糊。面部识别有用,但比较困难。背部号码好使。OCR已经很准了。
两个创新点:
1.定制了单模态类无关的重识别和检索CLIP目标
2.zero-shot能力和论文方法的区域重要特征
其它方法的问题:
- 仅限于预定义的类,需要团队标志服来追踪球员的身份。
- Siamese net work with triplet loss 无监督学习 来分开彼此
- 半交互,少了标注, transformer-based architecure
启发:
image encoder ~ vision transformer or resnet
text encoder ~ Transformer
consine similarity 计算相似度。
改造:
采样器不考虑同一批次中的一个运动员在另外一个运动员的实例图中可见,用label smooth的方式解决。Because we have pairs
of images and each pair is encoded by the same image encoder, we can encode both query and gallery images of the same batch at once. This doubles the effective batch size.
我们使用re排序[36]作为最终距离的后处理步骤矩阵,但也提供不重新排名。re-rank is prefer!!
Zero-shot Capacity: Zero shot 旨在仅根据描述学习类[37],从而允许在推理过程中检索新概念。
对选择的四个特征做消融实验:jersey number, jersey color, sex, skin color
关于soft label 本质是在one-hot上引入噪音,使得整体的loss下降。
Score-CAM算法来展示CLIP Vision Transformers在zero-shot下追踪球衣号码的能力。并通过ft model来可视化query和gallery images的相似性。
Score-CAM:是一种基于置信分数的视觉可解释性方法,它摆脱了对梯度的解释依赖。
关于它的讲解:https://zhuanlan.zhihu.com/p/329842645
算法关注到的区域
ref:
1.https://zhuanlan.zhihu.com/p/35040994 Siamese network 孪生神经网络
2.https://zhuanlan.zhihu.com/p/477760524 clip 介绍
3.论文:CLIP-ReIdent: Contrastive Training for Player Re-Identification https://arxiv.org/pdf/2303.11855.pdf 2023的顶会paper
4.code:https://github.com/DeepSportradar/2022-winners-player-reidentification-challenge
5.权重下载:https://drive.google.com/file/d/1Gm5J19okhLdnZTQLUsjfYoI0rwrLQ09i/view
更多推荐
所有评论(0)