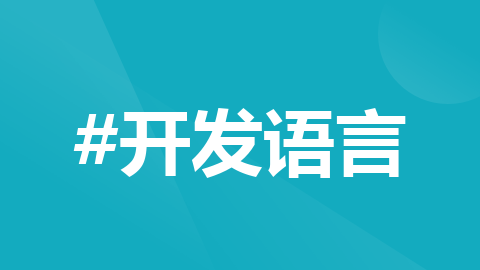
google-guava工具包常用工具类详解(持续更新)
Guava项目包含我们在基于Java的项目中所依赖的几个Google核心库:集合、缓存、原语支持、并发库、公共注释、字符串处理、I/O等等。这些工具中的每一个都被谷歌员工在生产服务中每天使用,也被许多其他公司广泛使用。
文章目录
一、google-guava工具包简介
1、概述
Guava项目包含我们在基于Java的项目中所依赖的几个Google核心库:集合、缓存、原语支持、并发库、公共注释、字符串处理、I/O等等。这些工具中的每一个都被谷歌员工在生产服务中每天使用,也被许多其他公司广泛使用。
2、引包
想要使用guava很简单,只需要引入依赖包就可以使用了:
<!-- maven -->
<!-- https://mvnrepository.com/artifact/com.google.guava/guava -->
<dependency>
<groupId>com.google.guava</groupId>
<artifactId>guava</artifactId>
<version>32.1.3-jre</version>
</dependency>
// gradle
// https://mvnrepository.com/artifact/com.google.guava/guava
implementation group: 'com.google.guava', name: 'guava', version: '32.1.3-jre'
二、新集合工具类
1、Multiset
Multiset 是 guava 包下一种新的集合,可以方便的统计集合中重复元素出现的次数
。
guava提供了许多Multiset:
HashMultiset::元素存放于 HashMap
LinkedHashMap:即元素的排列顺序由第一次放入的顺序决定,对应LinkedHashMap
TreeMultiset:元素被排序存放于TreeMap
EnumMultiset::元素必须是 enum 类型
ImmutableMultiset: 不可修改的 Mutiset,对应ImmutableMap
比如说,我们使用jdk实现一个单词在文档中出现的次数:
Map<String, Integer> counts = new HashMap<String, Integer>();
for (String word : words) {
Integer count = counts.get(word);
if (count == null) {
counts.put(word, 1);
} else {
counts.put(word, count + 1);
}
}
这很笨拙,容易出错,并且不支持收集各种有用的统计数据,比如总字数。
List<String> words = Arrays.asList("张三", "李四", "张三", "王五", "张三", "李四");
// 创建Multiset
Multiset<String> nameMultiset = HashMultiset.create();
// 添加
nameMultiset.addAll(words);
// 查询张三重复次数
System.out.println(nameMultiset.count("张三"));
// 给李四设置固定的次数
nameMultiset.setCount("李四", 10);
System.out.println(nameMultiset.count("李四"));
Multiset 接口中定义的方法主要有:
add(E element) :向其中添加单个元素
add(E element,int occurrences) : 向其中添加指定个数的元素
count(Object element) : 返回给定参数元素的个数
remove(E element) : 移除一个元素,其count值 会响应减少
remove(E element,int occurrences): 移除相应个数的元素
elementSet() : 将不同的元素放入一个Set中
entrySet(): 类似与Map.entrySet 返回Set<Multiset.Entry>。包含的Entry支持使用getElement()和getCount()
setCount(E element ,int count): 设定某一个元素的重复次数
setCount(E element,int oldCount,int newCount): 将符合原有重复个数的元素修改为新的重复次数
retainAll(Collection c) : 保留出现在给定集合参数的所有的元素
removeAll(Collectionc) : 去除出现给给定集合参数的所有的元素
2、Multimap
Multimap通常来说就是类似于Map<K, List<V>>或者Map<K, Set<V>>
,与Map不同的是,Multimap可以存放相同key的value值,并且将相同key的value值存放在集合中,我们可以这样理解Multimap:
存放:
a -> 1
a -> 2
a -> 4
b -> 3
c -> 5
结果:
a -> [1, 2, 4]
b -> [3]
c -> [5]
Multimap 实现类:
实现类 | key的行为 | value的行为 |
---|---|---|
ArrayListMultimap | HashMap | ArrayList |
HashMultimap | HashMap | HashSet |
LinkedListMultimap | LinkedHashMap | LinkedList |
LinkedHashMultimap | LinkedHashMap | LinkedHashMap |
TreeMultimap | TreeMap | TreeSet |
ImmutableListMultimap | ImmutableMap | ImmutableList |
ImmutableSetMultimap | ImmutableMap | ImmutableSet |
// ListMultimap
ListMultimap<String, Integer> treeListMultimap =
MultimapBuilder.treeKeys().arrayListValues().build();
// SetMultimap 创建key为int类型,value为枚举
// SetMultimap<Integer, MyEnum> hashEnumMultimap =
// MultimapBuilder.hashKeys().enumSetValues(MyEnum.class).build();
// 直接创建
Multimap multimap = ArrayListMultimap.create();
multimap.put("a",1);
multimap.put("a",2);
multimap.put("a",2);
multimap.put("b",3);
multimap.put("b",4);
System.out.println(multimap); // {a=[1, 2, 2], b=[3, 4]}
// 转为map :Map<K, Collection<V>>
Map map = multimap.asMap();
// 返回一个集合
Collection a = multimap.get("a");
// 所有value生成一个集合
Collection values = multimap.values();
// 是否包含
System.out.println(multimap.containsKey("a"));
3、BiMap
BiMap<K, V> 就是一个 Map<K, V>
,但是 它需要保证key和value都是唯一的,并且可以通过inverse()
方法转换为BiMap<V, K>
。
当需要维护 key和value双向映射时,我们通常会这样实现:
Map<String, Integer> nameToId = Maps.newHashMap();
Map<Integer, String> idToName = Maps.newHashMap();
nameToId.put("Bob", 42);
idToName.put(42, "Bob");
// 如果“Bob”或42已经存在,会发生什么?
// 如果我们忘记保持同步,就会出现奇怪的错误...
以上这样做法是有风险的。
BitMap有以下实现类:
HashBiMap:key和value都是HashMap
HashBiMap:key和value都是ImmutableMap
EnumBiMap:key和value都是EnumMap
EnumHashBiMap:key是EnumMap,value是HashMap
BiMap<String, Integer> bitMap = HashBiMap.create();
bitMap.put("a", 1);
bitMap.put("a", 2); // 重复的key会被替换
// 重复的value会抛异常:java.lang.IllegalArgumentException: value already present: 2
// bitMap.put("b", 2);
// 强行放,会删除以前的value
bitMap.forcePut("b", 2);
System.out.println(bitMap);
// 获取所有value
Set<Integer> values = bitMap.values();
// 所有key
Set<String> strings = bitMap.keySet();
// key和value倒置
BiMap<Integer, String> inverse = bitMap.inverse();
4、Table
Table类型其实就是Map<FirstName, Map<LastName, Person>>
,可以使用两个key对value进行索引。
Table的实现类:
HashBasedTable
:本质上是 HashMap<R, HashMap<C, V>>
TreeBasedTable
:本质上是TreeMap<R, TreeMap<C, V>>
ImmutableTable
ArrayTable
:它要求在构造时指定完整的行和列,但在表比较密集时,它由一个二维数组支持,以提高速度和内存效率。
Table的第一个key被设置为row的概念,第二个key被设置为column的概念,也就是说就像excel表一样,行和列才会对应一个值,相当于是一个二维平面。
Table<String, String, String> table = HashBasedTable.create();
table.put("张", "三", "居住山东");
table.put("张", "九", "居住北京");
table.put("王", "五", "居住山东");
// 返回 第二个key + value的map
Map<String, String> zhang = table.row("张");
System.out.println(zhang); // {三=居住山东, 九=居住北京}
// 返回 第一个key + value的map
Map<String, String> place = table.column("三");
System.out.println(place); // {张=居住山东}
Map<String, Map<String, String>> stringMapMap = table.rowMap();
System.out.println(stringMapMap); // {张={三=居住山东, 九=居住北京}, 王={五=居住山东}}
三、常用工具类
1、LoadingCache 缓存
LoadingCache 在实际场景中有着非常广泛的使用,通常情况下如果遇到需要大量时间计算或者缓存值的场景,就应当将值保存到缓存中。LoadingCache 和 ConcurrentMap 类似,但又不尽相同。
最大的不同是 ConcurrentMap 会永久的存储所有的元素值直到他们被显示的移除,但是 LoadingCache 会为了保持内存使用合理会根据配置自动将过期值移除。
LoadingCache 自己已经解决了缓存击穿问题
,同时获取一个key的value值时,如果该value不存在,会自动加锁,保证只会获取一次。
import com.google.common.cache.*;
import java.util.Arrays;
import java.util.concurrent.Callable;
import java.util.concurrent.ExecutionException;
import java.util.concurrent.TimeUnit;
public class CacheMain {
public static void main(String[] args) {
RemovalListener<String, String> removalListener = new RemovalListener<String, String>() {
public void onRemoval(RemovalNotification<String, String> removal) {
System.out.println("移出了key:" + removal.getKey());
System.out.println("移出了key:" + removal.getCause());
System.out.println("移出了key:" + removal.getValue());
}
};
// 定义缓存,key-value的形式
LoadingCache<String, String> caches = CacheBuilder
.newBuilder()
// 缓存最大值,超过最大值会移出
.maximumSize(10)
// 自上次读取或写入时间开始,10分钟过期
.expireAfterAccess(10, TimeUnit.MINUTES)
// 自上次写入时间开始,10分钟过期
.expireAfterWrite(10, TimeUnit.MINUTES)
// 基于权重驱逐key
.maximumWeight(100000)
.weigher(new Weigher<String, String>() {
public int weigh(String k, String v) {
// 获取权重
return v.getBytes().length;
}
})
// 设置移出监听器(同步的,高并发可能会阻塞)
.removalListener(removalListener)
// 构造获取缓存数据的方法
.build(
new CacheLoader<String, String>() {
public String load(String key) {
return createValue(key);
}
});
// 需要检查异常
try {
System.out.println(caches.get("key"));
} catch (ExecutionException e) {
e.printStackTrace();
}
// 不会检查异常
caches.getUnchecked("key");
caches.getIfPresent("key");
// 使用Callable,将call()方法的返回值作为缓存
try {
System.out.println(caches.get("ckey", new Callable<String>() {
@Override
public String call() throws Exception {
return "Callable";
}
})); // Callable
System.out.println(caches.get("ckey")); // Callable
} catch (ExecutionException e) {
e.printStackTrace();
}
// 直接放缓存
caches.put("key2", "v2");
// 将缓存作为ConcurrentMap输出
System.out.println(caches.asMap());
// 删除key
caches.invalidate("key");
caches.invalidateAll(Arrays.asList("key", "key1"));
caches.invalidateAll();
// 清除过期缓存,一般在写入的时候才会清理部分缓存,如果需要,可以手动清除一下
caches.cleanUp();
}
private static String createValue(String key) {
System.out.println("获取value");
return "value";
}
}
2、RateLimiter限流器
(1)基本使用
常用方法:
/**
* 创建一个稳定输出令牌的RateLimiter,保证了平均每秒不超过permitsPerSecond个请求
* 当请求到来的速度超过了permitsPerSecond,保证每秒只处理permitsPerSecond个请求
* 当这个RateLimiter使用不足(即请求到来速度小于permitsPerSecond),会囤积最多permitsPerSecond个请求
*/
public static RateLimiter create(double permitsPerSecond)
/**
* 创建一个稳定输出令牌的RateLimiter,保证了平均每秒不超过permitsPerSecond个请求
* 还包含一个热身期(warmup period),热身期内,RateLimiter会平滑的将其释放令牌的速率加大,直到起达到最大速率
* 同样,如果RateLimiter在热身期没有足够的请求(unused),则起速率会逐渐降低到冷却状态
*
* 设计这个的意图是为了满足那种资源提供方需要热身时间,而不是每次访问都能提供稳定速率的服务的情况(比如带缓存服务,需要定期刷新缓存的)
* 参数warmupPeriod和unit决定了其从冷却状态到达最大速率的时间
*/
public static RateLimiter create(double permitsPerSecond, long warmupPeriod, TimeUnit unit);
//每秒限流 permitsPerSecond,warmupPeriod 则是数据初始预热时间,从第一次acquire 或 tryAcquire 执行开时计算
public static RateLimiter create(double permitsPerSecond, Duration warmupPeriod)
//获取一个令牌,阻塞,返回阻塞时间
public double acquire()
//获取 permits 个令牌,阻塞,返回阻塞时间
public double acquire(int permits)
// 获取一个令牌,如果获取不到立马返回false
public boolean tryAcquire()
//获取一个令牌,超时返回
public boolean tryAcquire(Duration timeout)
获取 permits 个令牌,超时返回
public boolean tryAcquire(int permits, Duration timeout)
RateLimiter limiter = RateLimiter.create(2, 3, TimeUnit.SECONDS);
System.out.println("get one permit cost time: " + limiter.acquire(1) + "s");
System.out.println("get one permit cost time: " + limiter.acquire(1) + "s");
System.out.println("get one permit cost time: " + limiter.acquire(1) + "s");
System.out.println("get one permit cost time: " + limiter.acquire(1) + "s");
System.out.println("get one permit cost time: " + limiter.acquire(1) + "s");
System.out.println("get one permit cost time: " + limiter.acquire(1) + "s");
System.out.println("get one permit cost time: " + limiter.acquire(1) + "s");
System.out.println("get one permit cost time: " + limiter.acquire(1) + "s");
--------------- 结果 -------------------------
get one permit cost time: 0.0s
get one permit cost time: 1.331672s
get one permit cost time: 0.998392s
get one permit cost time: 0.666014s
get one permit cost time: 0.498514s
get one permit cost time: 0.498918s
get one permit cost time: 0.499151s
get one permit cost time: 0.488548s
因为RateLimiter滞后处理的,所以第一次无论取多少都是零秒
可以看到前四次的acquire,花了三秒时间去预热数据,在第五次到第八次的acquire耗时趋于平滑
(2)IP限流
import com.google.common.util.concurrent.RateLimiter;
import org.springframework.stereotype.Component;
import java.util.Map;
import java.util.concurrent.ConcurrentHashMap;
/**
* 限流工具
*/
@Component
public class LimiterClient {
/**
* key限流器 缓存
*/
private static final Map<String, RateLimiter> keyRateLimiterMap = new ConcurrentHashMap<>();
private RateLimiter getRateLimiterByKey(String key, double permitsPerSecond) {
return keyRateLimiterMap.computeIfAbsent(key, k -> RateLimiter.create(permitsPerSecond));
}
/**
* 获取ip限流器,1000秒限一次
*/
public RateLimiter getIpRateLimiter(String ip) {
return getRateLimiterByKey(ip, 0.001);
}
/**
* 获取全局限流器,每秒2000次
*/
private static final RateLimiter globalRateLimiter = RateLimiter.create(2000);
public RateLimiter getGlobalRateLimiter() {
return globalRateLimiter;
}
}
3、EventBus事件总线
EventBus是Guava的事件处理机制,是设计模式中的观察者模式(生产/消费者编程模型)的优雅实现。对于事件监听和发布订阅模式,EventBus是一个非常优雅和简单解决方案,我们不用创建复杂的类和接口层次结构。
(1)基本使用
import com.google.common.eventbus.EventBus;
import com.google.common.eventbus.Subscribe;
public class EventBusMain {
/**
* 定义消息实体,EventBus只支持一个消息参数,多个参数需要自己封装为对象
*/
public static class MyEvent {
private String message;
public MyEvent(String message) {
this.message = message;
}
public String getMessage() {
return message;
}
}
/**
* 定义消费者
*/
public static class EventListener {
// 使用@Subscribe 可以监听消息
@Subscribe
public void handler(MyEvent event) {
System.out.println("消费者接收到消息:" + event.getMessage());
}
}
public static void main(String[] args) {
// 定义EventBus
EventBus eventBus = new EventBus("test");
// 注册消费者
EventListener listener = new EventListener();
eventBus.register(listener);
// 发送消息
eventBus.post(new MyEvent("消息1"));
eventBus.post(new MyEvent("消息2"));
eventBus.post(new MyEvent("消息3"));
}
}
结果:
消费者接收到消息:消息1
消费者接收到消息:消息2
消费者接收到消息:消息3
(2)多消费者
在消费者的方法中,会自动根据参数的类型,进行消息的处理。
/**
* 定义消费者
*/
public static class EventListener {
// 使用@Subscribe 可以监听消息
@Subscribe
public void handler1(MyEvent event) {
System.out.println("消费者接收到MyEvent1消息:" + event.getMessage());
}
@Subscribe
public void handler2(MyEvent event) {
System.out.println("消费者接收到MyEvent2消息:" + event.getMessage());
}
@Subscribe
public void handler3(String event) {
System.out.println("消费者接收到String消息:" + event);
}
@Subscribe
public void handler4(Integer event) {
System.out.println("消费者接收到Integer消息:" + event);
}
}
public static void main(String[] args) {
// 定义EventBus
EventBus eventBus = new EventBus("test");
// 注册消费者
EventListener listener = new EventListener();
eventBus.register(listener);
// 发送消息
eventBus.post(new MyEvent("消息1"));
eventBus.post("消息2");
eventBus.post(99988);
}
结果:
消费者接收到MyEvent2消息:消息1
消费者接收到MyEvent1消息:消息1
消费者接收到String消息:消息2
消费者接收到Integer消息:99988
(3)DeadEvent
/**
* 如果没有消息订阅者监听消息, EventBus将发送DeadEvent消息
*/
public static class DeadEventListener {
// 消息类型必须是DeadEvent
@Subscribe
public void listen(DeadEvent event) {
System.out.println(event);
}
}
public static void main(String[] args) {
// 定义EventBus
EventBus eventBus = new EventBus("test");
// 注册消费者
EventListener listener = new EventListener();
eventBus.register(listener);
eventBus.register(new DeadEventListener()); // 注册DeadEventListener
// 发送消息
eventBus.post(new MyEvent("消息1"));
eventBus.post("消息2");
eventBus.post(99988);
eventBus.post(123.12D); // 如果发送的消息,没有消费者,就会到DeadEvent
}
执行结果
消费者接收到MyEvent2消息:消息1
消费者接收到MyEvent1消息:消息1
消费者接收到String消息:消息2
消费者接收到Integer消息:99988
DeadEvent{source=EventBus{test}, event=123.12}
(4)AsyncEventBus异步消费
import com.google.common.eventbus.AsyncEventBus;
import com.google.common.eventbus.Subscribe;
import java.util.concurrent.Executors;
public class EventBusMain2 {
/**
* 定义消费者
*/
public static class EventListener {
// 使用@Subscribe 可以监听消息
@Subscribe
public void handler1(String event) {
System.out.println("消费者1接收到String消息:" + event + ",线程号:" + Thread.currentThread().getId());
}
@Subscribe
public void handler2(String event) {
System.out.println("消费者2接收到String消息:" + event + ",线程号:" + Thread.currentThread().getId());
}
}
public static void main(String[] args) {
// 定义EventBus,消费者消费是异步消费
AsyncEventBus eventBus = new AsyncEventBus("test", Executors.newFixedThreadPool(10));
// 注册消费者
EventListener listener = new EventListener();
eventBus.register(listener);
// 发送消息
System.out.println("发送消息,线程号:" + Thread.currentThread().getId());
eventBus.post("消息2");
}
}
执行结果:
发送消息,线程号:1
消费者2接收到String消息:消息2,线程号:21
消费者1接收到String消息:消息2,线程号:22
4、BloomFilter布隆过滤器
import com.google.common.hash.BloomFilter;
import com.google.common.hash.Funnels;
/**
* 测试布隆过滤器(可用于redis缓存穿透)
*
*/
public class TestBloomFilter {
private static int total = 1000000;
private static BloomFilter<Integer> bf = BloomFilter.create(Funnels.integerFunnel(), total);
// private static BloomFilter<Integer> bf = BloomFilter.create(Funnels.integerFunnel(), total, 0.001);
public static void main(String[] args) {
// 初始化1000000条数据到过滤器中
for (int i = 0; i < total; i++) {
bf.put(i);
}
// 匹配已在过滤器中的值,是否有匹配不上的
for (int i = 0; i < total; i++) {
if (!bf.mightContain(i)) {
System.out.println("有坏人逃脱了~~~");
}
}
// 匹配不在过滤器中的10000个值,有多少匹配出来
int count = 0;
for (int i = total; i < total + 10000; i++) {
if (bf.mightContain(i)) {
count++;
}
}
System.out.println("误伤的数量:" + count);
}
}
5、Interner对字符串加锁
这样一个场景:lock是一个订单号,这个订单号比较特殊,对这个订单相关的操作必须串行,其他的订单可以并行执行。在多线程情况下,我们就需要对这个特殊的订单号进行加锁,以保证对这个订单操作的串行化,但是我们每一个进来的请求(线程)肯定带的订单号(lock)都是不一样的对象,如果贸然synchronized(lock)操作,肯定就会出现上边第一种情况的问题,所以这个时候Interners和intern方法就有用了。
jdk使用字符串.intern
方法,如果用多了会导致内存溢出。可以用以下方案:
(其实这种方式也有弊端,如果此时恰好执行了gc,会导致锁丢失,出现并发问题。或许使用缓存工具,通过对key设置过期时间,可以实现对字符串更友好的加锁)
import com.google.common.collect.Interner;
import com.google.common.collect.Interners;
import java.util.Date;
public class InternsTest {
private static final Interner<String> pool = Interners.newWeakInterner();
public static void main(String[] args) throws InterruptedException {
// s1+s2是两个不同的字符串对象,并不会存在于字符串常量池中
String s1 = "a";
String s2 = "b";
T1 t1 = new T1(s1 + s2);
T1 t2 = new T1(s1 + s2);
new Thread(() ->{
try {
m1(t1);
} catch (InterruptedException e) {
e.printStackTrace();
}
}).start();
m1(t2);
Thread.sleep(10000);
}
/**
* 加锁
*/
public static void m1(T1 t1) throws InterruptedException {
System.out.println(Thread.currentThread().getName() + "进来了" + new Date());
// 对特定字符串加锁
synchronized (pool.intern(t1.getId())) {
System.out.println(Thread.currentThread().getName() + "获取到锁" + new Date());
Thread.sleep(2000);
}
System.out.println(Thread.currentThread().getName() + "结束了" + new Date());
}
}
class T1{
public T1(String id) {
this.id = id;
}
private String id;
public String getId() {
return id;
}
public void setId(String id) {
this.id = id;
}
}
参考资料
https://www.cnblogs.com/guanbin-529/p/13022610.html
官网:https://github.com/google/guava
官方文档:https://github.com/google/guava/wiki
更多推荐
所有评论(0)